AI Banking: Best and Worst Use Cases
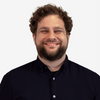
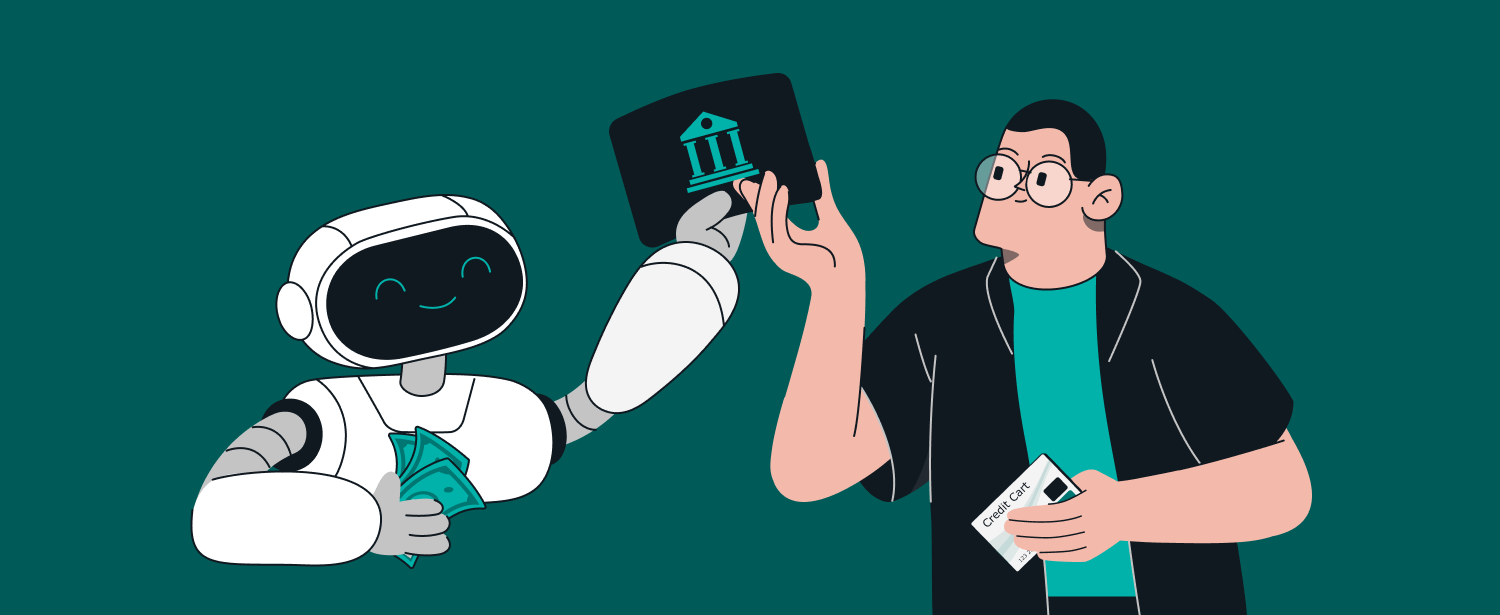
The banking industry is undergoing a tectonic shift, driven by the transformative power of Artificial Intelligence (AI).
From streamlined customer experiences to sophisticated fraud detection, AI promises to revolutionize how we bank. However, like any powerful tool, its potential for good is matched by its potential for pitfalls.
Our aim is to provide you with the expert insights and critical perspectives you need to navigate this rapidly evolving terrain. By understanding the strengths and weaknesses of AI in banking, you can form an informed opinion on its use and impact on the industry and contribute to its responsible implementation for a fairer and more secure financial future.
Stay tuned as we explore:
- AI's transformative potential: Streamlined processes, personalized experiences, and enhanced security.
- The dark side of AI: Algorithmic bias, discrimination, privacy concerns, and the risks associated with erroneous data outputs.
- Finding the sweet spot: Responsible AI implementation for maximum benefit and minimal harm.
- The future of AI in banking: Shaping a technology-driven industry with human values at its core.
Get ready for the exploration of AI in banking. Let's unlock its potential for good while vigilantly guarding against its unintended consequences.
AI in Banking
AI implementation has transitioned from a futuristic goal to a present-day reality. This transformative wave of AI is not limited to one segment of the banking "office" but extends across the front, middle, and back offices. Whether it's leveraging complex machine learning to combat money laundering or utilizing AI-powered customer service chatbots, the impact of AI is pervasive in the banking landscape.
Integrating AI in banking has streamlined finance-related processes, making them more personalized, insightful, and expeditious. Tasks that once took hours for human employees to complete can now be accomplished in minutes or even seconds with the assistance of AI.
While the banking industry has a longstanding reliance on technology and data, the advent of new data-enabled AI technology has elevated the potential for innovation to unprecedented levels. AI stands poised to enhance efficiency, facilitate a growth agenda, differentiate services, address risk and regulatory requirements, and positively impact the customer experience. The development of sophisticated AI systems, once deemed expensive and limited to specific use cases like high-frequency trading, is transforming. Deloitte's recent survey indicates that barriers to adoption, particularly related to cost and technology, are diminishing, making it increasingly feasible to implement and integrate AI technologies.
Organizations strategically invest in key areas such as cloud computing, big data platforms, and data applications with updated architecture, including microservices and event hubs. This approach minimizes upfront capital investments typically associated with the development, deployment, and scaling of AI solutions.
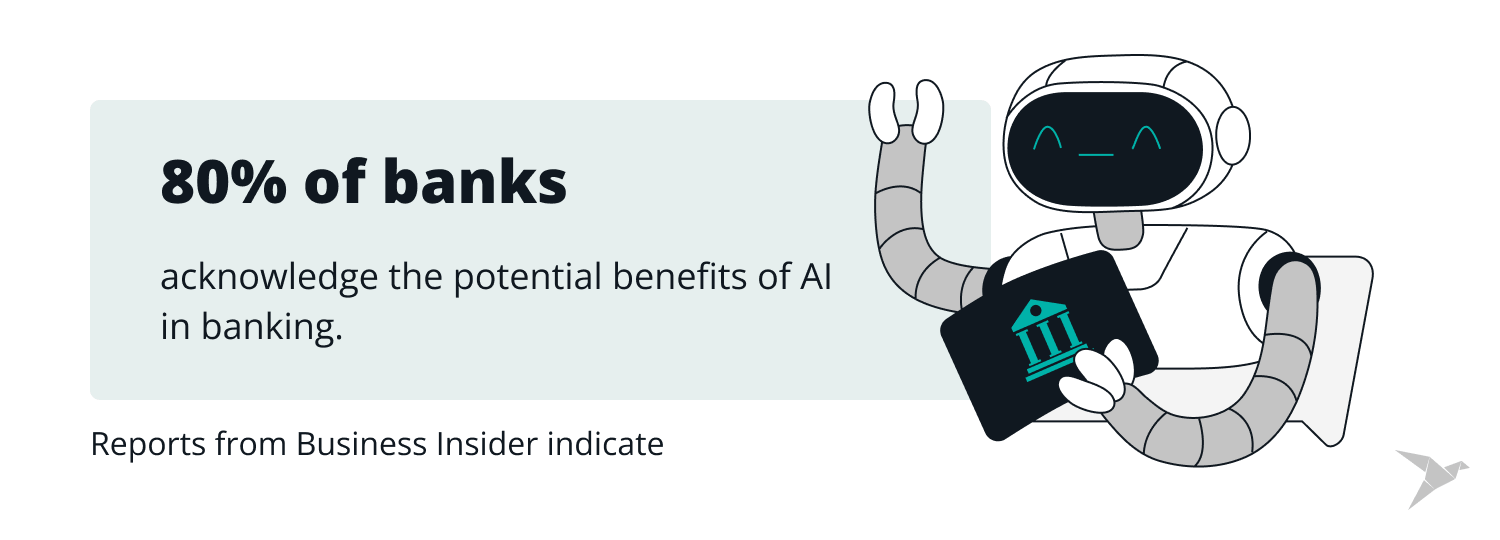
- Reports from Business Insider indicate that approximately 80% of banks acknowledge the potential benefits of AI in banking.
- According to Accenture, AI can automate up to 80% of routine banking tasks, potentially saving the industry $1 trillion globally by 2025.
- The AI in banking industry is poised for continued growth, with projections indicating a staggering reach of $64.03 billion by 2030.
- A McKinsey global survey reveals that approximately 60% of companies leverage AI in at least one business function.
- Juniper Research estimates that AI-powered fraud detection solutions will prevent $24.8 billion in global losses by 2025.
- According to the Cambridge Centre for Alternative Finance (CCAF), 56% of institutions have already integrated AI into risk management, with others actively incorporating AI into various business processes.
However, the question arises: How are these institutions incorporating AI, and what are the benefits and challenges associated with using artificial intelligence in banking and finance?
Best Use Cases of AI in Banking
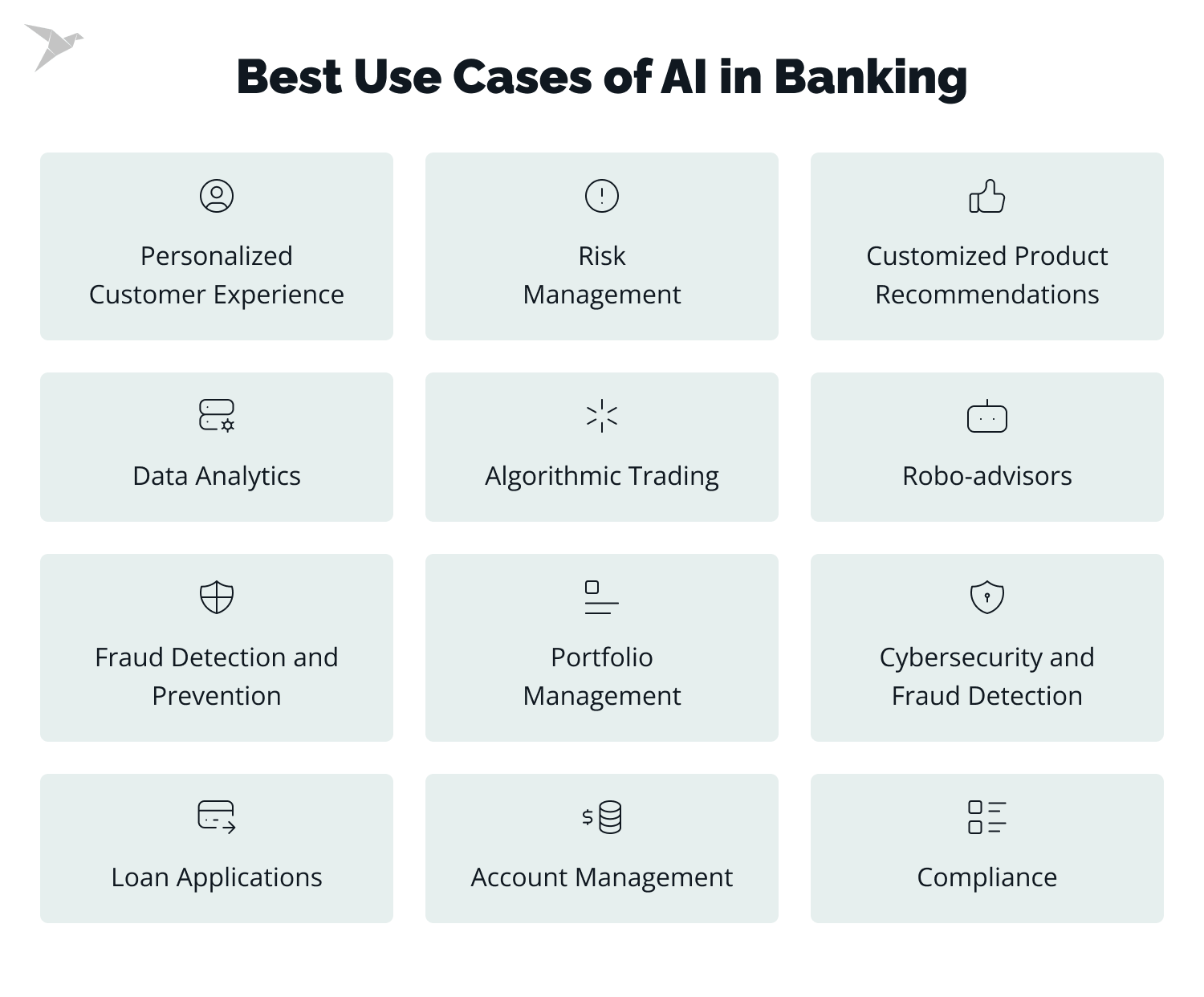
Here, we explore some of the best use cases of AI in banking, showcasing its ability to enhance customer engagement and satisfaction.
Personalized Customer Experience
The introduction of AI-driven chatbots marks a significant leap in customer interaction capabilities. These intelligent systems leverage Large Language Models and machine learning algorithms to engage customers in dynamic, personalized conversations.
- AI-driven chatbots analyze customer queries in real-time, understand contextual nuances, and provide tailored responses. From account inquiries to transaction details, chatbots seamlessly handle a myriad of tasks, offering immediate and personalized assistance.
- AI algorithms continuously learn from customer interactions, identifying patterns and preferences. This data is then utilized to generate predictive models, offering tailored financial recommendations, whether investment opportunities, customized banking products, or suitable loan options.
Customized Product Recommendations
AI algorithms analyze vast customer data, including transaction history, browsing behavior, and demographics, to generate personalized product recommendations. By understanding individual preferences and financial needs, banks can tailor their product offerings to match customer expectations and promote cross-selling opportunities.
Whether it's suggesting relevant banking products, investment opportunities, or loan options, AI-driven recommendation engines empower customers to make informed financial decisions aligned with their unique goals and aspirations. Through personalized product recommendations, banks can deepen customer relationships, increase customer lifetime value, and drive revenue growth while delivering exceptional value and relevance to their clientele.
Erica
A noteworthy illustration of AI-powered chatbots is Erica, the virtual assistant deployed by the Bank of America. Erica adeptly manages tasks related to credit card debt reduction and card security updates, handling over 50 million client requests in 2019. This exemplifies the impactful integration of AI in the banking sector for enhanced customer service and operational efficiency.
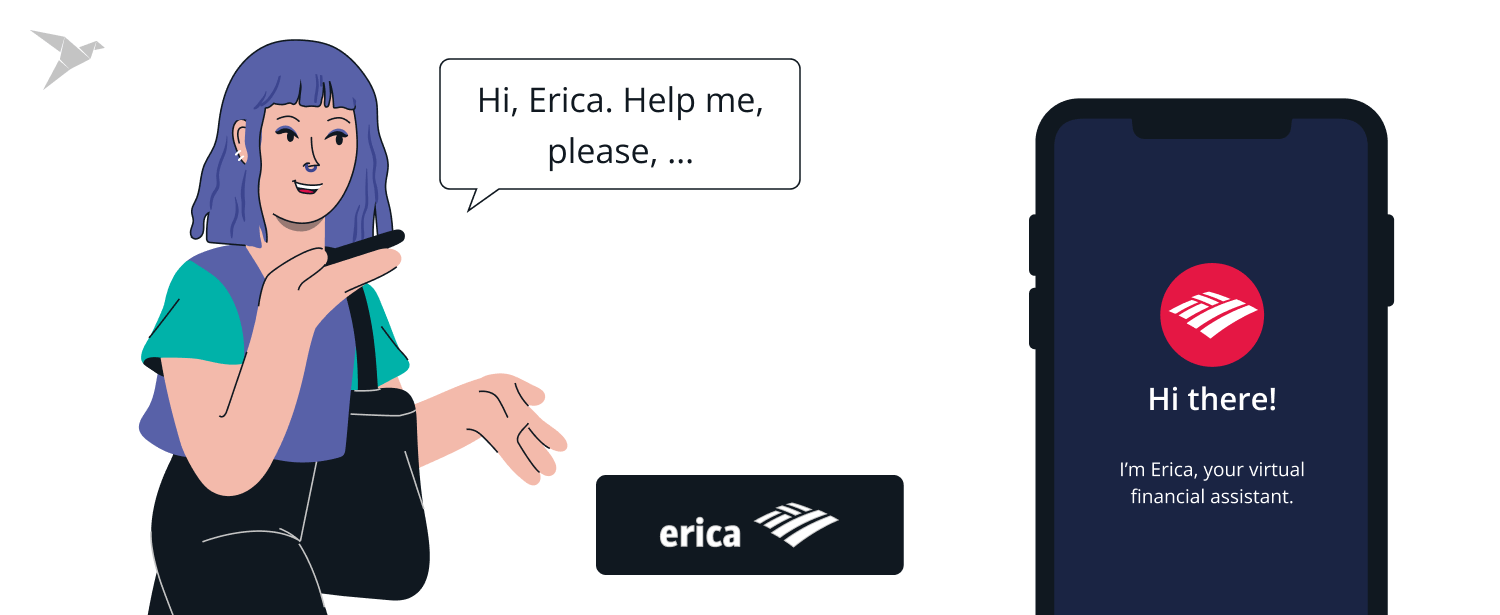
Risk Management
Predicting and mitigating risks before they materialize is a cornerstone of prudent risk management. AI-powered predictive analytics leverages advanced algorithms to analyze vast datasets, identify patterns, and forecast potential risks with unprecedented accuracy.
These AI algorithms continuously learn from historical data, market trends, and external factors to generate predictive models. By recognizing subtle patterns and correlations, AI enables financial institutions to foresee potential risks and take proactive measures.
AI introduces automation to this process, allowing for swift identification of potential risks and implementing targeted mitigation strategies. Automated risk assessment systems utilize AI algorithms to evaluate complex data sets, quickly identifying risk factors and their potential impact. These systems can then autonomously trigger predefined mitigation strategies or alert risk management teams for further analysis and action.
Fraud Detection and Prevention
Real-time fraud analysis enables immediate intervention and prevents unauthorized transactions, safeguarding both the financial institution and its customers. The continuous learning aspect ensures that the system evolves to counter emerging fraud techniques effectively.
Behavioral Biometrics and Anomaly Detection:
- Traditional authentication methods are no longer sufficient in the face of sophisticated cyber threats. AI introduces behavioral biometrics and anomaly detection to add an extra layer of security.
- Behavioral biometrics analyze individual user behavior patterns, such as typing speed, device interaction, and navigation habits. Anomaly detection algorithms then raise alerts when deviations from these patterns occur.
Vectra's Cognito technology stands at the forefront of identifying and pursuing cyber threats. This automated software streamlines threat detection, accelerates event investigations, and identifies compromised data, especially vital for financial institutions facing targeted cyber threats.
Data Analytics
As millennials increasingly turn to the stock market for investment opportunities, the demand for AI-driven apps facilitating effective navigation of the stock market has surged. Numerous smartphone apps now leverage AI to analyze historical and current data about businesses and their stocks.
AI's contribution to data collection and analysis is instrumental in making informed decisions. AI algorithms process massive volumes of data, extracting valuable insights that guide data-driven decision-making. This capability helps financial institutions predict demand, analyze market trends, and improve customer experiences through personalized services.
Automated data collection and analysis are multifaceted benefits of AI in banking and finance. Artificial Intelligence efficiently performs these processes, processing massive datasets and extracting valuable insights.
Perfios, an Indian business, offers an effective data analytics platform utilized by banks and non-bank financial institutions. It aids in fraud prevention, supports better loan selections, facilitates asset management, and provides reliable credit scores. Major companies such as Deutsche Bank, Canara HSBC, and Home Credit Finance trust Perfios, which has garnered over $120 million in investments.
Algorithmic Trading
AI-driven algorithms analyze vast datasets swiftly, identify market trends, and execute trades with split-second timing. This automated approach mitigates the risks associated with human emotions in trading, ensuring data-driven insights guide financial decisions for maximum returns and minimized losses.
HDFC Bank and Renaissance Technologies LLC, a New York-based hedge fund, exemplify the successful use of algorithmic trading in the financial sector. Renaissance Technologies' Medallion Fund, with average annual returns of 66% since its inception in 1988, underscores the effectiveness of AI-driven strategies in financial markets.
Robo-advisors
By automating and simplifying back-end office tasks, robo-advisors enable humans to concentrate on more strategic and creative activities. These AI-based fintech solutions redefine investment strategies.
Wealthfront, a California-based automated investment company, exemplifies the potential of robo-advisors. Offering assistance in goal planning, transparency in portfolio building, and a range of account services, Wealthfront has reported assets under management of around $20 billion as of September 2019, solidifying its position as a noteworthy player in the robo-advisory space.
Portfolio Management
Wealth and portfolio management witness a significant transformation by incorporating AI in the banking and finance sector. This technological advancement brings financial services to users' fingertips, especially beneficial for those who cannot frequent physical bank visits. AI-powered technologies efficiently manage banking services and enhance mobile banking operations.
When users initiate digital transactions through banking apps, AI applications actively track and send immediate transaction alerts in case of any suspicious activities, ensuring secure and monitored transactions. Additionally, AI plays a pivotal role in automating the debit/credit card management system, streamlining the authentication process and enhancing the safety of transactions. Thus, AI systems contribute to the advancement of secure and efficient mobile banking services.
Compliance
Manual compliance processes are time-consuming and require substantial investments. Moreover, the dynamic nature of regulatory changes demands constant updates to internal processes. AI and ML in banking utilize deep learning and Natural Language Processing (NLP) to read and interpret new compliance requirements swiftly. While AI doesn't replace compliance analysts, it significantly accelerates their operations, ensuring efficiency in navigating complex regulatory landscapes.
Loan Applications
Artificial Intelligence and machine learning find their most promising application in the banking sector, particularly in loan applications. The AI algorithm's prowess lies in evaluating a customer's repayment ability and their likelihood to devise plans for debt settlement. The demand for AI-powered credit scoring solutions is substantial, given the considerable population worldwide lacking traditional banking access, with only a fraction qualifying for credit.
AI-based loan systems go beyond traditional credit assessment by scrutinizing the behavior and patterns of customers with limited or poor credit history, effectively determining their creditworthiness.
Account Management
Artificial intelligence in banking can be harnessed to assist users in efficiently managing their accounts. For example, AI models can be trained to set up automatic payments, send timely notifications for impending payments, and facilitate changes in bank information. This user-friendly technology empowers clients to navigate account management tasks independently, reducing reliance on manual interventions by managers and enhancing overall efficiency.
Cybersecurity and Fraud Detection
AI-driven smart banking services are captivating service providers and customers alike by effectively detecting risks and minimizing fraudulent activities. AI technology within banking apps scans transactional data, identifying irregular user behavior patterns. By leveraging smart AI tools and apps, banking companies can fortify their defenses against potential breaches, ensuring the security of their business operations.
Danske Bank, Denmark's largest bank, is an exemplary case of utilizing AI for fraud detection. Implementing a fraud detection algorithm powered by deep learning, the bank experienced a 50% increase in fraud detection capabilities and a 60% reduction in false positives. The AI-driven system automated critical decisions while redirecting certain cases to human analysts for thorough scrutiny.
Worst Use Cases of AI in Banking
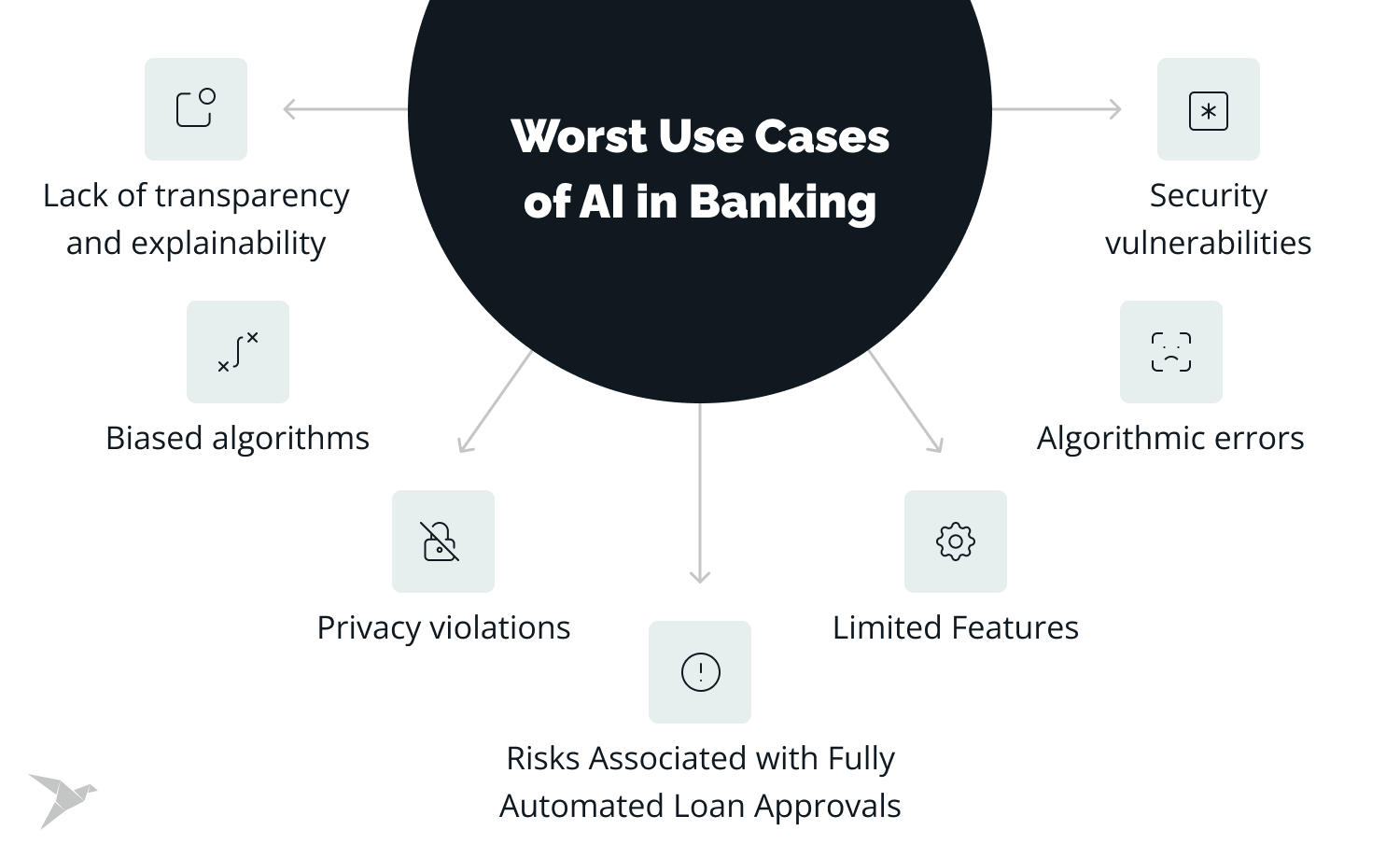
While AI has the potential to revolutionize banking, its misuse can lead to serious problems. Here are some of the worst potential use cases of AI in banking:
Biased algorithms
While AI promises a brighter future for banking, its potential can be eclipsed by a dark side: biased algorithms. This seemingly objective technology can perpetuate and amplify societal inequalities, leading to discriminatory lending practices, unfair insurance rates, and exclusion from financial services.
These are not hypothetical fears; they are real concerns with potentially devastating consequences. Biases can creep into AI algorithms in various ways:
- Data Bias: If the data used to train the algorithm is inherently biased, the resulting model will inevitably perpetuate those biases.
- Algorithmic Bias: Even with unbiased data, the design and structure of the algorithm can introduce unintended biases.
- Human Bias: The choices made by developers and data scientists can unconsciously introduce biases into the system.
The consequences of biased AI algorithms in banking are far-reaching:
- Denied access to loans, insurance, and other financial services can hinder economic mobility and perpetuate poverty.
- Unfair treatment can damage trust in banks and financial institutions, hindering financial inclusion and stability.
- AI bias can exacerbate existing social inequalities, widening the gap between privileged and disadvantaged communities.
Lack of transparency and explainability
Let's delve into the dangers lurking in the shadows of AI:
1. Black Box Decisions:
Many AI algorithms operate like black boxes, producing outputs without explaining the reasoning behind them. This lack of transparency creates problems:
- Unjust Outcomes: With understanding the decision-making process, potential biases in the algorithm can be protected, leading to discriminatory loan denials, unfair insurance rates, or biased investment recommendations.
- Loss of Trust: When customers are kept in the dark about how AI impacts their financial lives, trust erodes, hindering adoption and jeopardizing the potential benefits of AI.
- Regulatory Scrutiny: Opaque AI makes it difficult for regulators to assess compliance and mitigate potential risks, leading to increased scrutiny and potential regulatory backlash.
2. Algorithmic Bias:
AI algorithms are trained on data, and biases present in that data can be perpetuated and amplified by the algorithm, leading to:
- Discrimination: Biased algorithms can discriminate against individuals based on factors like race, gender, or income, leading to unfair outcomes.
- Loss of Opportunity: Lack of transparency makes it difficult to identify and address biases, denying individuals access to financial services or products.
- Erosion of Financial Inclusion: If AI perpetuates existing inequalities, it further hinders financial inclusion and widens the economic gap.
3. Accountability and Responsibility:
With AI, pinpointing responsibility for decisions becomes challenging:
- Unclear Liability: When issues arise, it's difficult to determine who is accountable: the developers, the bank, or the algorithm itself.
- Erosion of Human Oversight: If humans relinquish control over decisions to AI without understanding the reasoning, accountability becomes blurred.
- Reduced Consumer Protection: Consumers have limited recourse if an unexplained AI decision negatively impacts them.
Privacy violations
The more data collected, the higher the risk of breaches exposing sensitive financial information. Malicious actors can exploit AI systems to gain access to this treasure trove, leading to identity theft and financial losses. AI algorithms can create detailed profiles of individuals, potentially leading to discriminatory practices in loan approvals, insurance pricing, or even targeted marketing campaigns based on sensitive information.
The constant collection and analysis of data can create a sense of being watched, eroding our control over our financial information and privacy.
Security vulnerabilities
AI introduces a complex new attack surface for malicious actors. Unlike traditional software, AI systems are constantly evolving, learning, and adapting, making them challenging to monitor and secure. Here's how vulnerabilities can unfold:
- Poisoned Data: Attackers can inject biased or manipulated data into the training process, leading AI algorithms to make flawed decisions with potentially disastrous consequences. Imagine biased loan approvals or fraudulent transactions slipping through AI-powered security filters.
- Model Manipulation: Hackers can exploit weaknesses in AI models themselves, causing them to malfunction, misclassify data, or even provide false positives, opening doors for unauthorized access or financial manipulation.
- Adversarial Examples: Malicious actors can craft specifically designed inputs (e.g., fake images or voices) that trick AI systems, bypassing security systems and gaining access to sensitive information. Imagine bypassing facial recognition for ATM access or fooling voice authentication for phone banking.
Algorithmic errors
One of the most concerning is the potential for algorithmic errors. These errors, stemming from biases, data imbalances, and unforeseen complexities within AI algorithms, can have profound consequences for customers, erode trust, and hinder responsible innovation. Navigating this ethical minefield necessitates a nuanced understanding of the risks and a commitment to robust mitigation strategies.
Imagine a loan applicant, qualified and deserving, denied access to crucial credit due to biased data embedded in an AI-powered decision system. Or, consider discriminatory insurance rates dictated by opaque algorithms, exacerbating societal inequalities. These are not dystopian nightmares; they are real-world possibilities with severe implications:
- Perpetuation of Inequities: Biased algorithms can amplify existing societal biases, further marginalizing vulnerable populations and hindering financial inclusion.
- Erosion of Trust: Unfair and inexplicable outcomes erode customer trust in banks and the financial system, jeopardizing stability and growth.
- Reputational Damage: Public backlash and regulatory scrutiny in the wake of algorithmic errors can inflict significant reputational damage and financial losses on banks.
Limited Features
Limited features, particularly explainability and adaptability, create fertile ground for ethical concerns, potentially jeopardizing fairness, trust, and the very stability of the financial system.
Imagine these unsettling possibilities:
An AI-powered creditworthiness assessment system denies an individual loan access without clear explanation, perpetuating financial exclusion and fostering mistrust.
Unrecognized biases within training data embed themselves in AI models, leading to discriminatory outcomes in insurance pricing, wealth management, or other services.
Limited adaptability in AI systems renders them susceptible to manipulation by malicious actors, potentially jeopardizing client data and financial stability.
Limited features in AI can:
- If left unchecked, they can amplify existing societal biases, further marginalizing vulnerable populations and hindering economic inclusion.
- When customers feel unfairly judged by opaque algorithms, their faith in financial institutions wanes, potentially destabilizing the entire system.
- Public outcry and regulatory scrutiny in the wake of ethical missteps can severely damage bank reputations and financial well-being.
Risks Associated with Fully Automated Loan Approvals
While efficiency and accessibility are enticing propositions, the specter of ethical concerns, particularly around fully automated decisions, cannot be ignored. Let's delve into the potential pitfalls and chart a course for responsible innovation in this critical domain.
Imagine scenarios where:
- Loan applications are rejected without clear explanations, leaving individuals frustrated and potentially facing discriminatory outcomes.
- Algorithmic analysis, devoid of human understanding, overlooks an individual's unique circumstances, leading to unfair rejections despite their potential repayment ability.
- Overlooking individual financial vulnerability, AI systems might prioritize repayment metrics over individual well-being, pushing borrowers into unsustainable debt burdens.
- Unforeseen biases or vulnerabilities within AI models could trigger widespread loan defaults, jeopardizing financial stability.
How to Successfully Integrate AI
Define Clear Objectives and Use Cases
Begin by outlining specific objectives and identifying use cases where AI can deliver tangible value. Whether it's enhancing customer service, improving risk management, or streamlining back-office operations, clarity on goals is paramount. Define measurable success metrics to gauge the effectiveness of AI implementation.
Cultivate a Data-Driven Culture
AI thrives on data. Ensure access to high-quality, relevant data sources and establish robust data governance frameworks. Encourage a culture that values data-driven decision-making and fosters collaboration between data scientists, business analysts, and domain experts.
Invest in Talent and Expertise
Building AI capabilities requires a skilled workforce with expertise in data science, machine learning, and domain-specific knowledge. Invest in talent acquisition, training programs, and partnerships with academic institutions to nurture a pool of AI talent within the organization.
Prioritize Ethical AI Practices
Embed ethical considerations into the fabric of AI development and deployment. Ensure transparency, fairness, and accountability in AI algorithms to mitigate biases and uphold ethical standards. Adhere to regulatory guidelines and industry best practices to foster trust and confidence among stakeholders.
Prioritize Security and Privacy
Implement robust security measures and encryption protocols to safeguard sensitive data and protect against cybersecurity threats. Adhere to industry standards and regulatory requirements to ensure compliance with data privacy laws and regulations. Proactively monitor and mitigate risks to maintain trust and confidence among customers.
Conclusion
Foremost among these challenges is the paramount concern of safeguarding client data. Given the industry's unwavering commitment to the security of banking data and operations, integrating banking services with third-party AI solutions demands meticulous attention.
The selection of an AI-powered solution necessitates the expertise of a professional team well-versed in AI development. Furthermore, banks must ensure that the interface linking their apps with AI is not only efficient but also fortified with additional security measures, safeguarding both user data and financial information.
Another critical challenge lies in preparing the AI model to cater specifically to the intricacies of the banking industry. The unique terminology and context within the banking and finance sectors require meticulous training of the technology with pertinent and up-to-date data. Insufficient training may lead to the AI providing inadequate responses to user queries. To mitigate such scenarios, thorough preparation of AI for the nuances of fintech operations becomes imperative.
At TechMagic, we address these challenges through a comprehensive suite of AI development and integration services. Leveraging the most advanced AI models available, we seamlessly integrate them with our clients' services, automating processes and enhancing overall business outcomes.
Interested to learn more about TechMagic?
Contact usFAQs
-
In what ways can AI improve customer service in banking?
AI can enhance customer service by providing 24/7 support through chatbots, addressing inquiries, facilitating transactions, and offering personalized assistance. It streamlines processes, reduces response times, and ensures a seamless and efficient banking experience for customers.
-
What role does AI play in offering personalized banking experiences?
AI analyzes customer data to understand preferences, behavior, and financial patterns. This information enables customized services, such as tailored product recommendations, personalized financial advice, and targeted marketing. AI-driven personalization enhances customer satisfaction and loyalty.
-
What challenges exist regarding bias in AI-driven decision-making in banking?
Bias in AI models can perpetuate existing inequalities and result in discriminatory outcomes. In banking, biased decision-making may affect loan approvals, credit assessments, and other financial processes. Addressing bias requires ongoing efforts to identify, mitigate, and eliminate discriminatory patterns in AI algorithms.